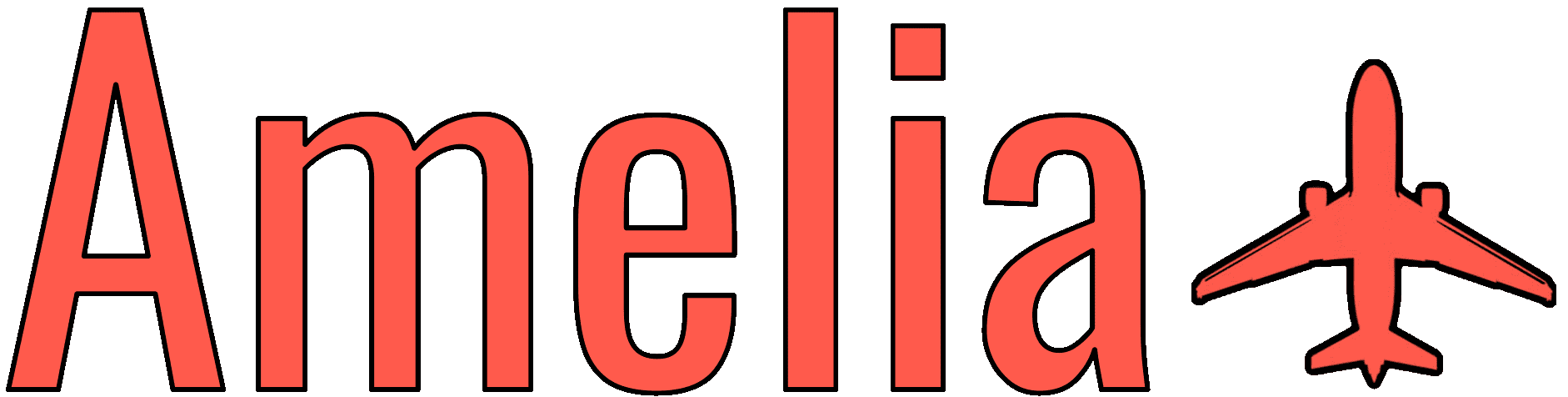
Our Motivation
The growing demand for air travel requires technological advancements in air traffic management as well as mechanisms for monitoring and ensuring safe and efficient operations.
Some trends…
A close call…
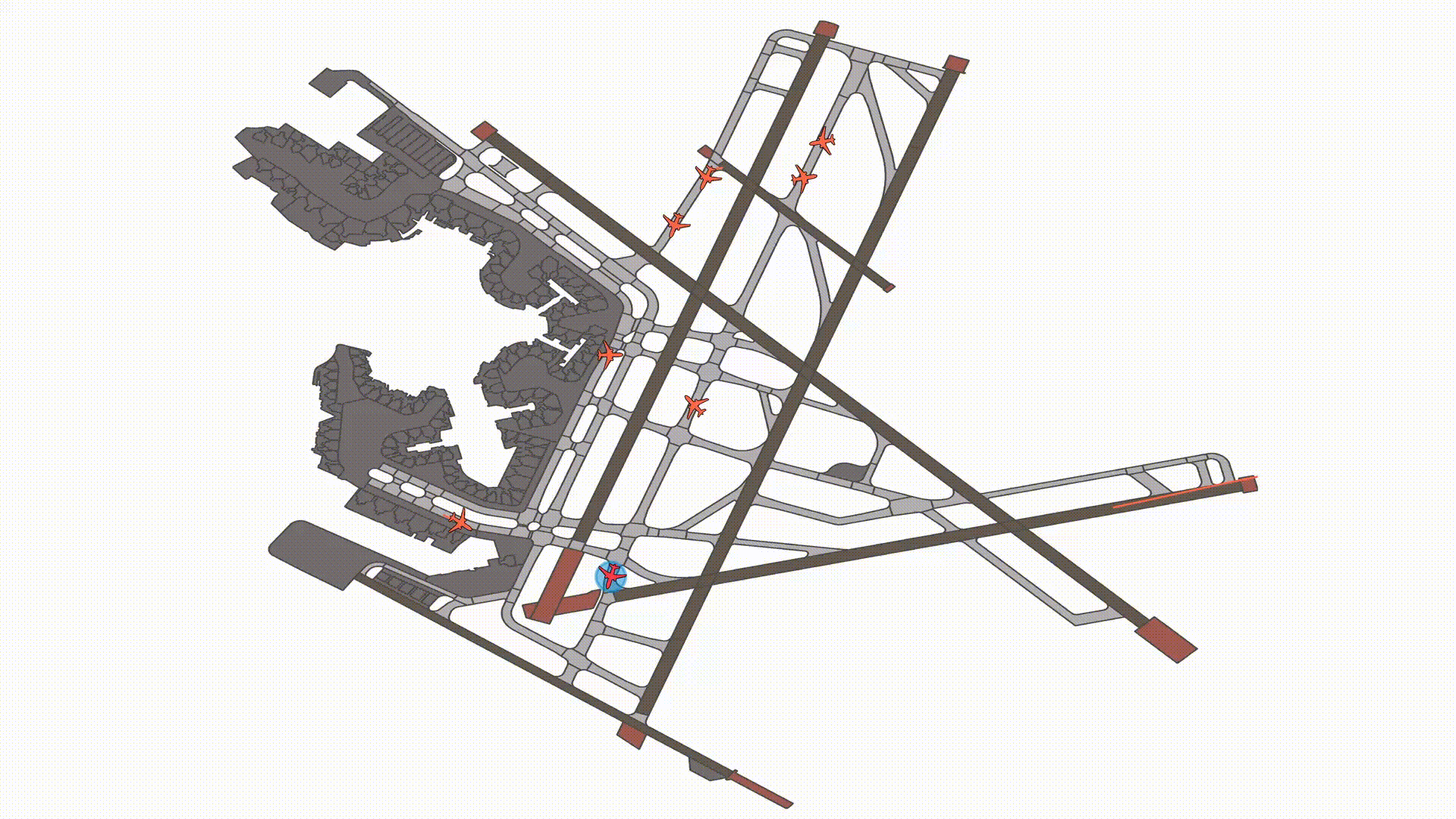
A runway incursion at Boston-Logan Intl. Airport (2x speed)
To improve airport safety and efficiency and encourage further research in this direction we introduce Amelia, a large-scale dataset of airport surface movement and a toolkit for data analysis, visualization, benchmarking, and behavior modeling.
Our dataset, Amelia-48 comprises more than a year’s worth of data collection across 48 airports and TRACON facilities within the US National Airspace System, which is ∼50TB of raw data.
Additionally, inspired by the success of motion prediction models in the AV domain for safety monitoring and multi-agent coordination, we introduce Amelia-TF, a transformer-based large multi-agent multi-airport trajectory forecasting model trained on 292 days or over 9B tokens of position data encompassing 10 different airports.
Keywords
Aviation | Machine Learning | Deep Learning | Data Science
Trajectory Prediction | Anomaly Detection | Safety
Our Framework
Our Code
Principal investigators
Team members
Collaborators
Papers
Amelia: A Large Model and Dataset for Airport Surface Movement Forecasting
Ingrid Navarro *, Pablo Ortega-Kral *, Jay Patrikar *, Haichuan Wang, Zelin Ye, Jong Hoon Park, Jean Oh and Sebastian Scherer
*Denotes equal contribution
@inbook{navarro2024amelia,
author = {Ingrid Navarro and Pablo Ortega and Jay Patrikar and Haichuan Wang and Zelin Ye and Jong Hoon Park and Jean Oh and Sebastian Scherer},
title = {AmeliaTF: A Large Model and Dataset for Airport Surface Movement Forecasting},
booktitle = {AIAA AVIATION FORUM AND ASCEND 2024},
chapter = {},
pages = {},
doi = {10.2514/6.2024-4251},
URL = {https://arc.aiaa.org/doi/abs/10.2514/6.2024-4251},
eprint = {https://arc.aiaa.org/doi/pdf/10.2514/6.2024-4251},
}